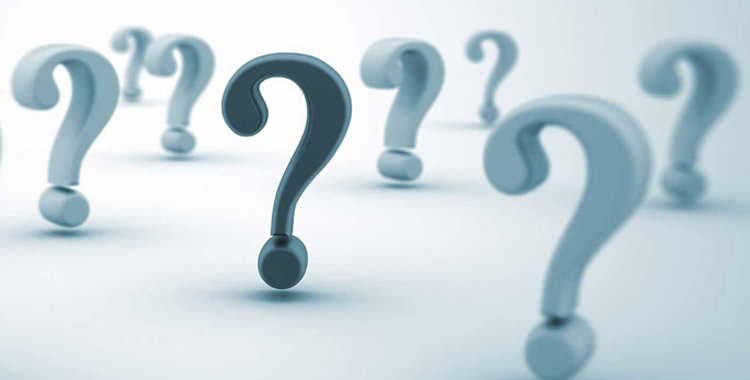
Community Data-Sharing 101: The Importance of the Question
This post is the first of a series from Dr. Alex Kolker, Community Impact Manager of United Way of the Quad Cities Area in Davenport, Iowa. Over the last three years, the Quad Cities has been using our Community Solutions Data Warehouse (CSDW) software to create a community-wide, multi-partner, multi-disciplinary data warehouse. The project links student achievement data from eight different school districts – in two states – with outcomes data from local non-profits and post-secondary achievement data from the National Student Clearinghouse. In his blog entries, Dr. Kolker will explain, step by step, the level of planning and partnership-building necessary to create a project on this scale.
—
Ten years ago, the organized pooling and analysis of data took place only in academia and government agencies. Now, in the civic and nonprofit worlds – thanks in part to multi-agency partnerships such as those of Promise Neighborhoods and Strive, and to new buzz words such as “data-driven decision-making” – everyone seems to be warehousing data (or, at the very least, talking about warehousing data). It seems very much like a fad – just the latest flavor of the month.
I disagree. Collective data analysis, when it’s done right, is such a powerful tool that fifty years from now everyone will be doing it. It’s the “doing it right” part that’s the real trick.
The difference between (a) creating a data-sharing project “because everyone’s doing it” and (b) creating a data-sharing project that will actually change the way your organization does business is all in the planning you undertake to put your project together.
Many communities are tempted, right from the start, to amass all of the data available. However, too much data very quickly turns into data overload, leading to your project losing focus. It is better to have no data at all than to have millions of data points sitting in a mainframe somewhere that no one ever accesses. We all have statistical reports in our filing cabinets that we never even looked at, let alone needed.
Instead, the first step for a well-designed data warehouse project is to decide why you want to pool together data. What question are you trying to answer? It is easy to say, “We want to create a data warehouse to track economic stability in our community.” But dig deeper: what parameter/population/time frame do you want information about? For example: “In our community, how strong is the correlation between a person’s educational attainment and his or her annual earnings?” This level of specificity will dictate which data you need to collect and which partners you will need to bring to the table.
And don’t stop there. Whenever possible, try to pair your data with demographic, income, and/or geographic data. This will allow your data-mining an extra level of granularity: not just how strong the correlation between educational attainment and annual income is, but whether this correlation is stronger or weaker in different sub-populations.
Say, for example, that in one neighborhood 95% of high school dropouts are living below the poverty line, but in the neighborhood next door only 50% of high school dropouts live in poverty. This becomes more than just an interesting stat. There are all sorts of reasons why this might be the case:
- There are more employment opportunities for high-school dropouts in Neighborhood 2.
- There is some social condition (for example: high crime, lack of bus route access) in Neighborhood 1 that is blocking economic growth.
- There is some additional resource – a workforce training program, for example – in Neighborhood 2 that is responsible for this change. (If so, replicate the program in Neighborhood 1 and then use your data collection to track the outcomes of your new program.)
This one data finding has just opened up a whole realm of possibilities for identifying and addressing specific community needs.
This is where real “data-driven decision-making” begins.
Community Data-Sharing 101 Blog Series
- The Importance of the Question
- General Warehouse Design
- Partner Engagement
- Building Partnerships
- Picking a Data Steward Partner
- Demystifying the Data-Sharing Agreement
- The Data-Sharing Agreement as a Living Document
To learn more about the Quad Cities’ educational initiatives and how nFocus Solutions is impacting the community, read the case study, The Quad Cities: Making Shared Data a Reality.